Security Best Practices for AI Innovation
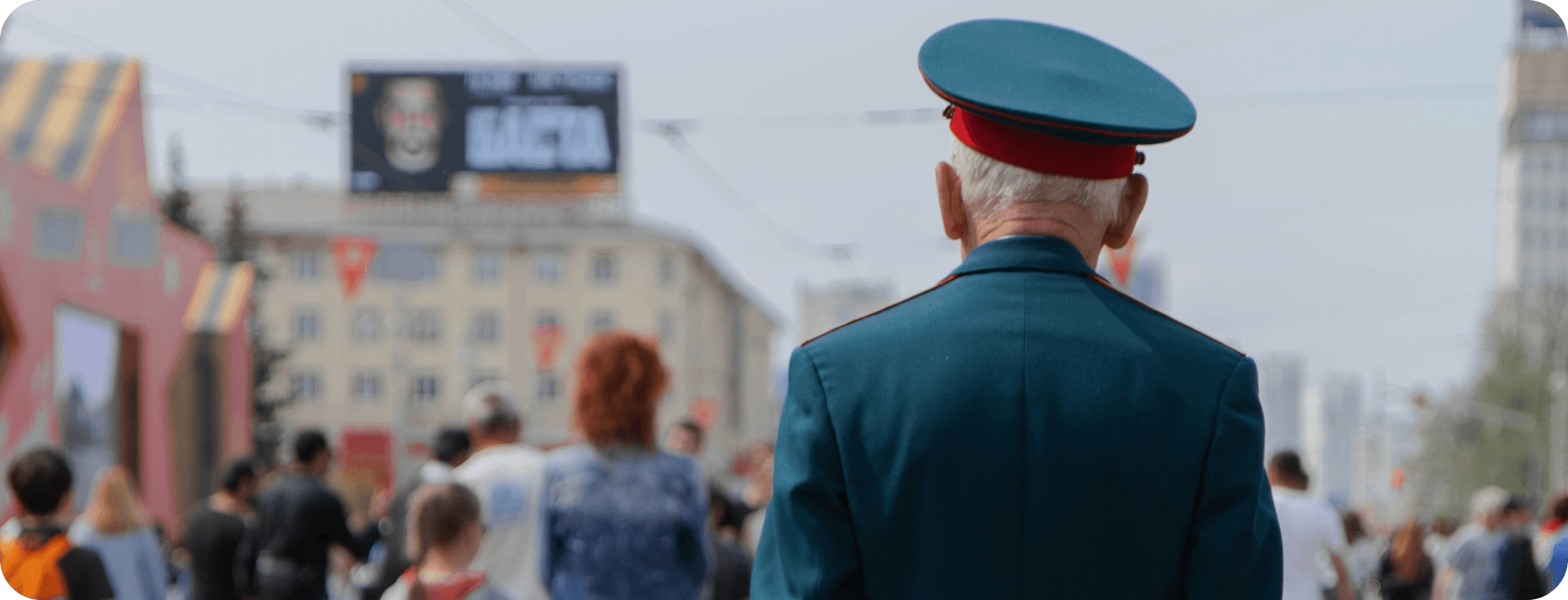
Historical Context
The historical context of AI security intertwines with the evolution of artificial intelligence and its increasing integration into various facets of enterprise operations. AI technologies, initially seen as futuristic concepts, began gaining traction in the late 20th century. However, it wasn't until the early 21st century that AI's rapid adoption brought about a significant transformation in both technological and cybersecurity landscapes. By the late 2010s, AI had become integral to modern development processes, workload automation, and big data analytics, fundamentally reshaping industries and services, including banking and customer service[1].
The proliferation of AI led to heightened awareness of the security implications associated with its use. During the 2010s, discussions around AI often centered on ethical considerations and the potential for AI to replace human jobs. Yet, the security risks inherent in AI adoption gradually emerged as a critical area of concern. In this period, threat actors began leveraging AI to perpetrate cyberattacks, using sophisticated methods to dispense malware and compromise code and datasets[2].
As AI technologies became more pervasive, the need for robust AI security measures became evident. By 2022, the adoption of AI had surged by 250% compared to 2017, according to McKinsey, underscoring the urgency for addressing AI-related vulnerabilities[3]. The security landscape faced new challenges, such as data breaches facilitated by AI vulnerabilities and the manipulation of AI models through techniques like data poisoning and prompt injections[4].
Concurrently, the cybersecurity sector began harnessing AI to combat these threats. The market for AI in cybersecurity is projected to grow significantly, emphasizing the reliance on AI to identify and mitigate large-scale cyberattacks. This development underscores the dual role of AI as both a tool for innovation and a vector for potential security threats, necessitating the evolution of cybersecurity frameworks and standards to keep pace with AI advancements[3][4].
Common Security Challenges in AI
Artificial Intelligence (AI) systems are increasingly integrated into various sectors, yet they face several common security challenges that need to be addressed to ensure their safe and ethical use.
Adversarial Attacks
Adversarial attacks involve manipulating input data to deceive AI systems into making incorrect predictions or decisions. These attacks exploit vulnerabilities in AI models by introducing subtle changes to the input data, causing misinterpretation and undesired outputs[5]. To counter adversarial attacks, AI systems should incorporate adversarial training, robust input validation, and anomaly detection mechanisms to recognize and resist manipulation[5].
Data Breaches
Data breaches are a major security concern for AI systems as they often manage vast amounts of sensitive information. Compromised data storage or transmission channels can result in unauthorized access to confidential data, violating privacy laws and potentially leading to severe financial and reputational damage for organizations[5]. To mitigate these risks, AI systems must adopt robust encryption methods and secure communication protocols, coupled with regular security audits and compliance with data protection regulations like GDPR and CCPA[5].
Bias and Discrimination
AI systems can inadvertently perpetuate or amplify biases present in their training data, leading to discriminatory outcomes in critical areas such as hiring, lending, and law enforcement[5]. Addressing bias requires diverse and representative training data, fairness-aware algorithms, and regular audits of AI systems for biased outcomes[5]. Organizations should establish ethical guidelines and oversight mechanisms to ensure AI applications operate fairly and transparently[5].
Model Theft
Model theft, or model inversion, occurs when attackers recreate an AI model by extensively querying it to approximate its functionality. This can lead to intellectual property theft and misuse of the model's capabilities[5]. Preventing model theft involves limiting the information inferred from model outputs and using techniques like differential privacy to obscure underlying data[5]. Implementing strict access controls and monitoring usage patterns can also help detect unauthorized attempts to extract model details[5].
Manipulation of Training Data
Data poisoning, or manipulation of training data, involves injecting malicious data into the training dataset to influence AI model behavior. This can degrade performance or cause biased predictions[5]. Protecting against data poisoning requires strict data curation and validation processes, as well as regular audits to identify and remove malicious inputs. Robust learning algorithms that resist outliers can enhance resistance to data manipulation[5].
Resource Exhaustion Attacks
Resource exhaustion attacks, such as denial-of-service (DoS) attacks, aim to overwhelm AI systems by consuming computational resources, disrupting services, and degrading performance[5]. Mitigating these attacks involves implementing rate limiting, resource allocation controls, and employing load balancing mechanisms to ensure the system remains operational under high demand[5]. Regular monitoring and anomaly detection can help identify and respond to such attacks promptly[5].
Security Best Practices
As artificial intelligence (AI) becomes increasingly integrated into organizational processes, ensuring its secure deployment and usage is paramount. This section outlines best practices for securing AI systems across their development and operational lifecycle, emphasizing the need for robust security measures, data protection, and compliance with evolving regulations.
Integrating Security Throughout the AI Lifecycle
One of the foundational steps in securing AI systems is integrating security practices at every stage of the AI lifecycle. This involves employing secure coding practices, regularly scanning for vulnerabilities, conducting thorough code reviews, and performing both static and dynamic analysis [6]. These measures ensure that security is not an afterthought but a core component of AI development.
To bolster AI system security, organizations are encouraged to appoint a responsible individual, typically the Chief Information Security Officer (CISO), to oversee AI cybersecurity. This role involves ensuring a secure deployment environment characterized by strong governance, solid architecture, and secure configurations [6]. Additionally, creating a threat model to guide security best practices is essential. This model helps in assessing threats and planning mitigations effectively, whether developed in-house or provided by vendors [6].
Data Privacy and Leakage Prevention
AI systems require access to vast amounts of data, which necessitates stringent privacy standards to protect sensitive information. Organizations should establish clear guidelines that dictate ethical data usage, obtaining proper consent, and anonymizing data when necessary [6]. Implementing robust data governance frameworks not only safeguards user privacy but also enhances trust in AI systems.
A proactive approach to data leakage involves recognizing the risks associated with AI, such as unintentional data transmission, and employing strategies like developing custom front-ends and using sandboxes to control data flow and mitigate bias [6]. Training employees about the risks of AI technology and instituting a comprehensive workforce training program can further reduce cybersecurity risks associated with "shadow IT" [6].
Ensuring Compliance with Regulations
Adopting AI securely and compliantly requires organizations to establish clear boundaries and responsible AI policies. Regular data audits can reveal how data is collected and stored, highlighting areas for improvement and ensuring compliance with privacy-by-design principles [6]. As AI-specific laws and regulations evolve, organizations must stay informed about new requirements, such as the European Union's Artificial Intelligence Act, which categorizes AI applications by risk and imposes stricter controls on high-risk applications [6].
By integrating these best practices, organizations can effectively manage AI risks, protect sensitive data, and ensure compliance with regulatory standards, ultimately fostering a secure and innovative AI environment.
Industry Standards and Regulations
In the realm of artificial intelligence (AI) innovation, adhering to industry standards and regulations is crucial to ensuring ethical and secure practices. The Federal Trade Commission (FTC) has underscored the importance of model-as-a-service companies honoring their privacy commitments and prohibiting the use of customer data for undisclosed purposes[7]. Failure to comply can lead to severe repercussions, such as the deletion of unlawfully obtained data and models, thereby highlighting the high stakes for enterprises using AI tools, particularly those built on large language models (LLMs) or extensive datasets[7].
A pivotal concern in this landscape is the risk of data breaches, which can expose confidential customer information and result in significant liability for organizations[7]. Moreover, the potential for employees or customers to unintentionally input confidential data into generative AI tools raises further risks of data exposure, necessitating robust safeguards to mitigate legal repercussions and protect reputational integrity[7]. It is considered unfair or deceptive in the United States for companies to adopt permissive data practices, such as sharing consumer data with third parties or using it for AI training, without transparent and upfront communication with consumers[7]. Retroactive amendments to terms of service or privacy policies without informing consumers of such changes can lead to severe legal consequences[7].
In parallel, ethical considerations play a vital role in technology, fostering trust and confidence among users while ensuring the responsible handling of personal data[8]. For instance, the European Union's General Data Protection Regulation (GDPR) is a comprehensive data protection law designed to enhance privacy and data protection by giving individuals more control over their data and setting strict consent requirements[8]. This regulation exemplifies how standards and regulations aim to protect individuals' rights and promote responsible data handling.
Additionally, ethical issues in IT, such as privacy and data protection, emphasize the importance of robust security measures, informed consent, and transparency in privacy policies[8]. Organizations that prioritize ethical practices can build trust, prevent bias and discrimination, and contribute to a sustainable digital landscape[8]. Therefore, as AI continues to evolve, aligning innovation with ethical standards and regulatory compliance is essential to maintaining accountability and integrity in the industry[8].
Case Studies
Autonomous Vehicles
Autonomous vehicles represent a compelling case study in AI innovation, highlighting the multifaceted security and ethical challenges associated with AI systems. These vehicles rely on complex algorithms to interpret data from sensors, make real-time decisions, and interact with other vehicles and road users. One major concern is the inconclusive evidence generated by the inferential statistics and machine learning techniques these algorithms employ. While they produce probable knowledge, the inherent uncertainty poses risks, especially in critical scenarios such as pedestrian detection or obstacle avoidance[9]. The difficulty in scrutinizing how these systems draw conclusions—known as the inscrutable evidence problem—further complicates the issue, as the algorithms' decision-making processes are often opaque to users and regulators[10].
Facial Recognition Technology
Facial recognition technology (FRT) serves as another example of AI innovation facing security and ethical scrutiny. The potential for bias and discrimination in FRT is significant, as these systems may reflect and perpetuate pre-existing social values and biases[11]. Concerns about unjustified actions arise when decisions based on FRT do not account for the broader societal implications, such as potential violations of privacy or the impact on marginalized communities. The opacity of FRT algorithms exacerbates these issues, as affected individuals may not understand how their data is being used or how decisions are made[12]. This lack of transparency highlights the importance of incorporating ethical considerations into the design and deployment of FRT systems[12].
AI in Healthcare
AI applications in healthcare, such as predictive analytics and personalized medicine, illustrate both the transformative potential and the ethical challenges of AI. These systems can provide actionable insights to improve patient outcomes but also raise issues of inscrutable and misguided evidence when the data used is flawed or biased[11]. The opacity of AI systems in healthcare can lead to autonomy concerns, as patients may not fully understand or consent to how their data is utilized in treatment decisions[12]. Additionally, the challenge of traceability emerges in determining responsibility and accountability when AI-driven decisions lead to adverse outcomes[10]. Therefore, robust security and ethical frameworks are essential to harness the benefits of AI in healthcare while mitigating associated risks[9].
Future Trends
The future of AI innovation is poised to be shaped by several key trends that align with security best practices. As AI technologies continue to evolve, their integration into business processes will demand heightened attention to security, fairness, and transparency.
One significant trend is the rise of Generative AI, which has seen widespread adoption across various industries, promising to enhance productivity and efficiency [13]. This trend is likely to continue as companies invest in developing more advanced AI frameworks and models. However, this growth must be balanced with robust AI risk management to ensure that AI implementations meet organizational standards and contribute to overall success [13].
Another future trend is the emphasis on AI risk management frameworks that prioritize robustness, fairness, and data security [13]. As AI systems become more integrated into critical applications, ensuring their reliability against adversarial attacks and unexpected conditions will be essential [13]. Organizations are expected to invest in enhancing model generalization, adversarial training, and continuous monitoring to mitigate such risks effectively.
The demand for explainability and transparency in AI systems is also anticipated to grow. As AI decisions increasingly impact diverse stakeholders, developing tools to interpret and explain AI processes will become a priority [13]. This shift will likely lead to innovations in model interpretability and the creation of more transparent AI systems, fostering trust and accountability.
Finally, privacy and data security will remain at the forefront of AI innovation trends. As AI systems handle more sensitive data, organizations will need to implement stricter data minimization techniques, anonymization, and decentralized learning models to protect personal information [13]. This focus on data security will be crucial in maintaining compliance with data protection laws and regulations.
In conclusion, as AI continues to evolve, addressing its security challenges is crucial for fostering a secure and innovative digital landscape.

Start Your Cybersecurity Journey Today
Gain the Skills, Certifications, and Support You Need to Secure Your Future. Enroll Now and Step into a High-Demand Career !
Fusion Cyber Blogs
RECENT POSTSU.S. Coast Guard's New Cyber Rule: What Maritime Firms Must Know
The U.S. Coast Guard's new cybersecurity rule, effective July 16, 2025, mandates stricter cyber protections for maritime organizations, including risk assessments, incident response plans, and a designated Cybersecurity Officer.
Read MoreCurrent State of Federal Cybersecurity
The current state of federal cybersecurity is shaped significantly by recent initiatives and directives aimed at bolstering the United States' cyber defenses. A pivotal element in this effort is President Biden's Executive Order 14028, which underscores the urgent need to improve the nation's cybersecurity posture in response to increasingly sophisticated cyber threat
Read More